Real-time Neural Dense Elevation Mapping for Urban Terrain with Uncertainty Estimations
Accepted by IEEE Robotics and Automation Letters
Having good knowledge of terrain information is essential for improving the performance of various downstream tasks on complex terrains, especially for the locomotion and navigation of legged robots.
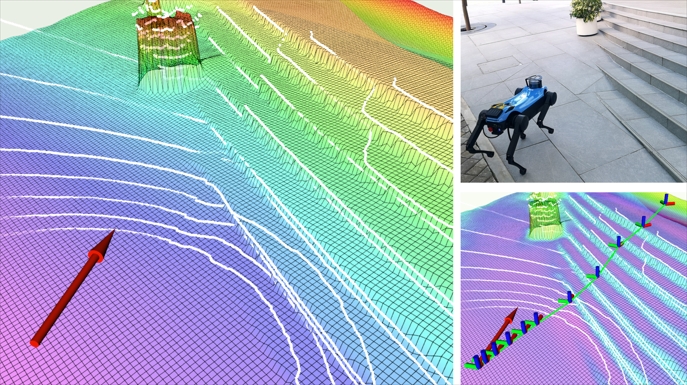
We present a novel framework for neural urban terrain reconstruction with uncertainty estimations. It generates dense robot-centric elevation maps online from sparse LiDAR observations.
Each point cloud frame is pre-processed to obtain frame features which are then used to update a group of statistical point features as the model input. Our pre-processing approach achieves high computational efficiency and high robustness to noise when integrating multiple point frames. A generative Bayesian model recovers the terrain features based on the prior knowledge of urban terrains, where an edge generation branch is jointly trained for better learning performance. By introducing the concept of Bayesian learning, our model simultaneously returns dense reconstruction uncertainty estimates that reflect the model’s confidence in the mapping results.
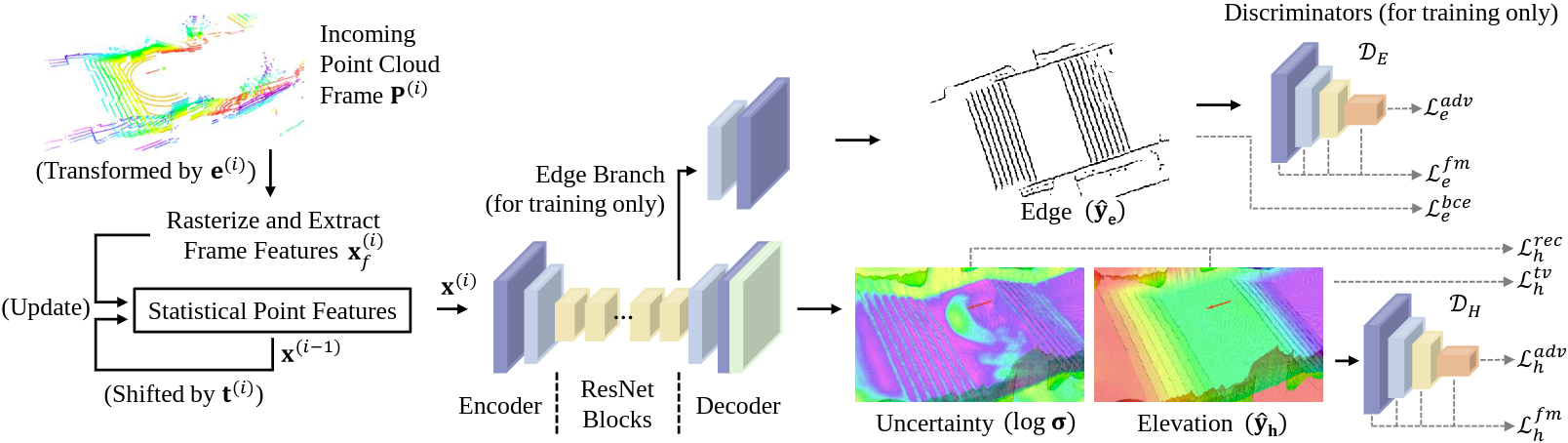
Qualitative results show that our approaches provide accurate dense elevation maps with high reconstruction quality and can recover detailed terrain structures even under noisy and sparse observations. By integrating uncertainty values in the reconstruction loss, the mapping performance is improved in height accuracy and edge sharpness. In addition, providing point feature representations can further assist to recover the terrain features and improves the mapping quality.
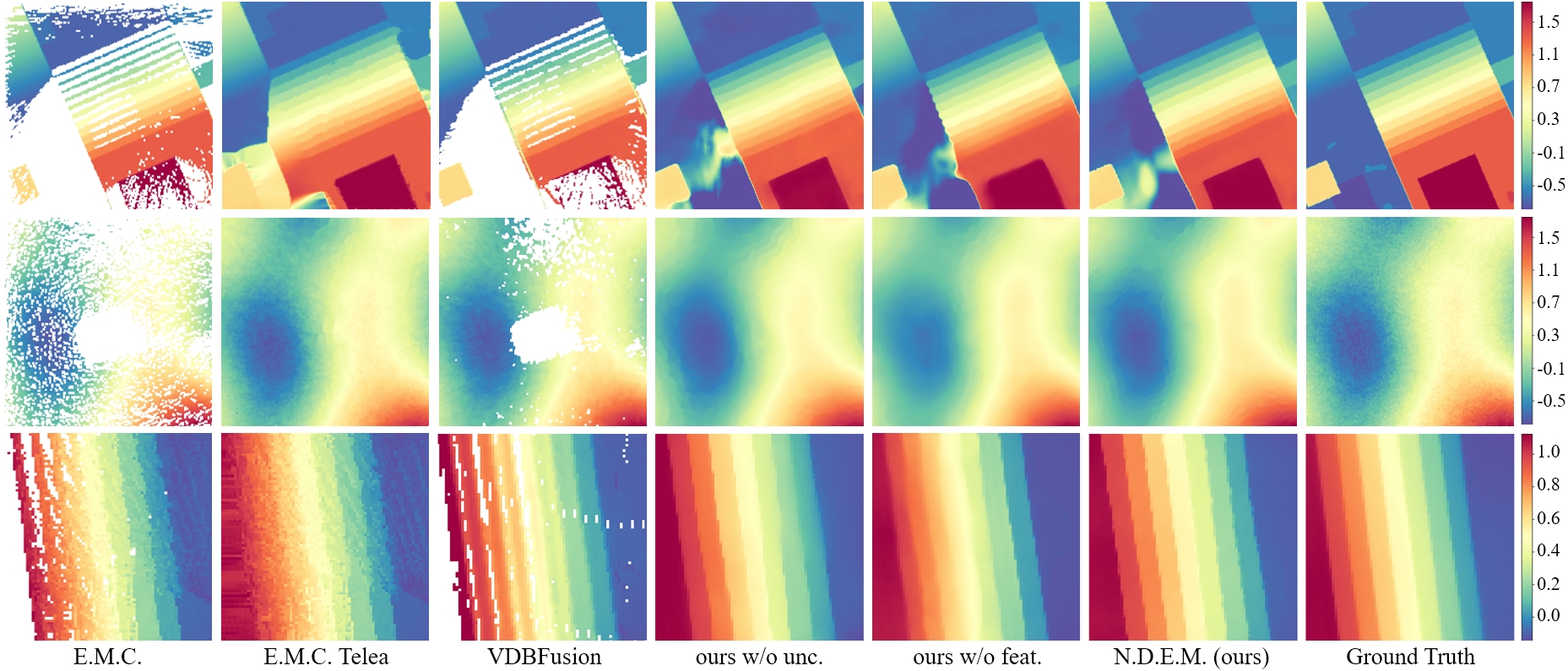
To intuitively compare the performance of existing online elevation mapping approaches, we conduct continuous mapping experiments on real-world stairs. Our framework maintains high mapping quality when the robot approaches and walks up the stairs, while other methods fail to recover detailed stair structures.
With strong capabilities to build urban terrains at a glance, our approach further assists the navigation and exploration tasks to rapidly find feasible paths even with extremely sparse observations.
By returning higher uncertainty values at stair edges and occluded regions, our approach informs the downstream tasks of the potential dangers resulting from the mapping inaccuracy.
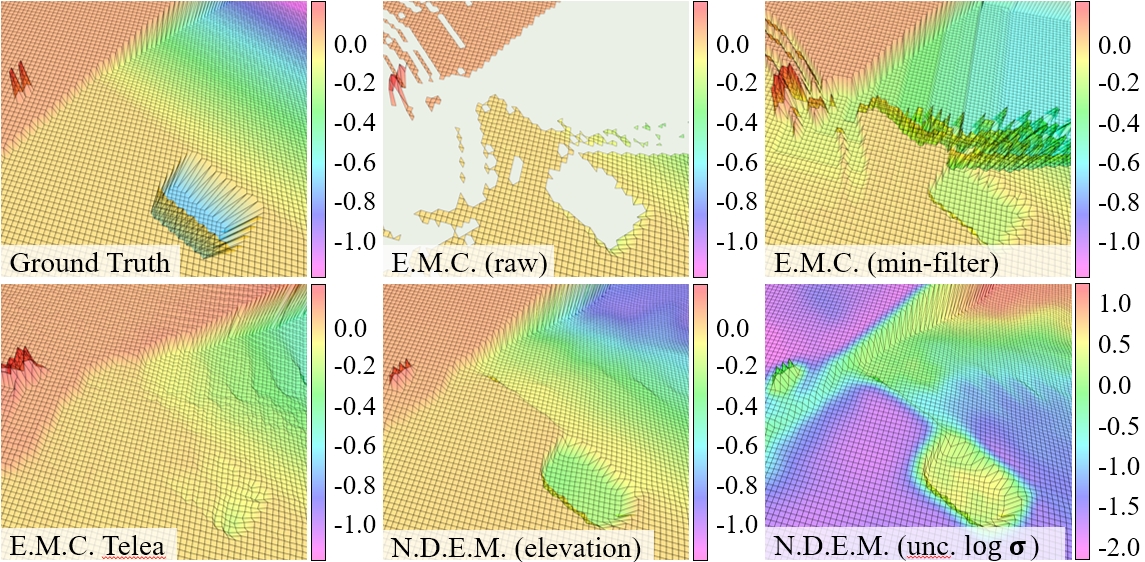
The uncertainty estimations further reflect the confidence of our model on the generated maps in the relationship between the percentage (orange bar plot) of the grids with different levels of error and the corresponding estimated uncertainty values (purple curve).
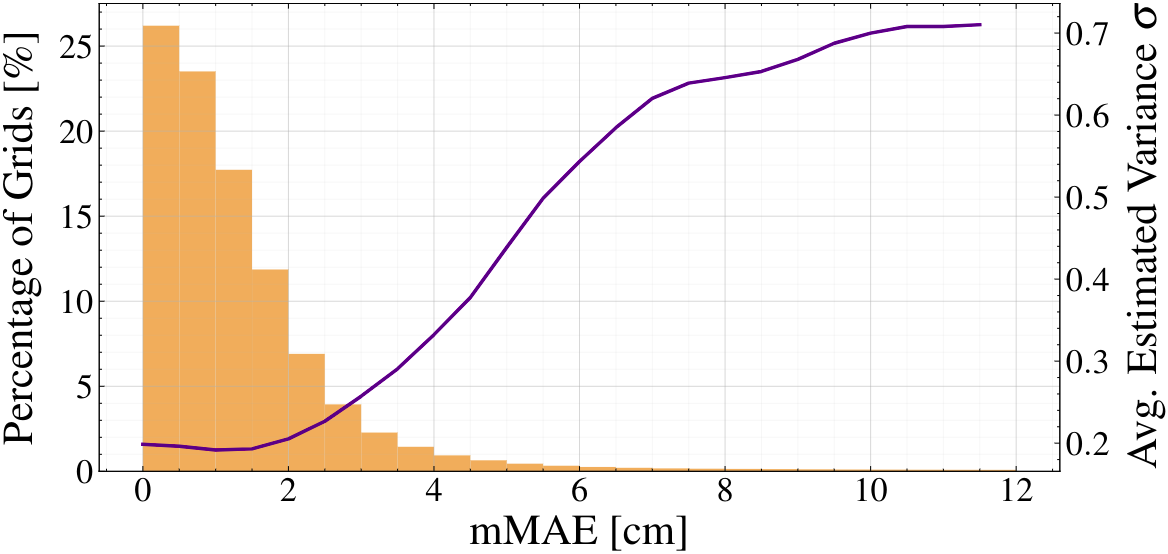
As shown in the tables, our approach provides more accurate map results in height values and their gradients both in simulations and real-world experiments while maintaining high representation fidelity and structural similarity.
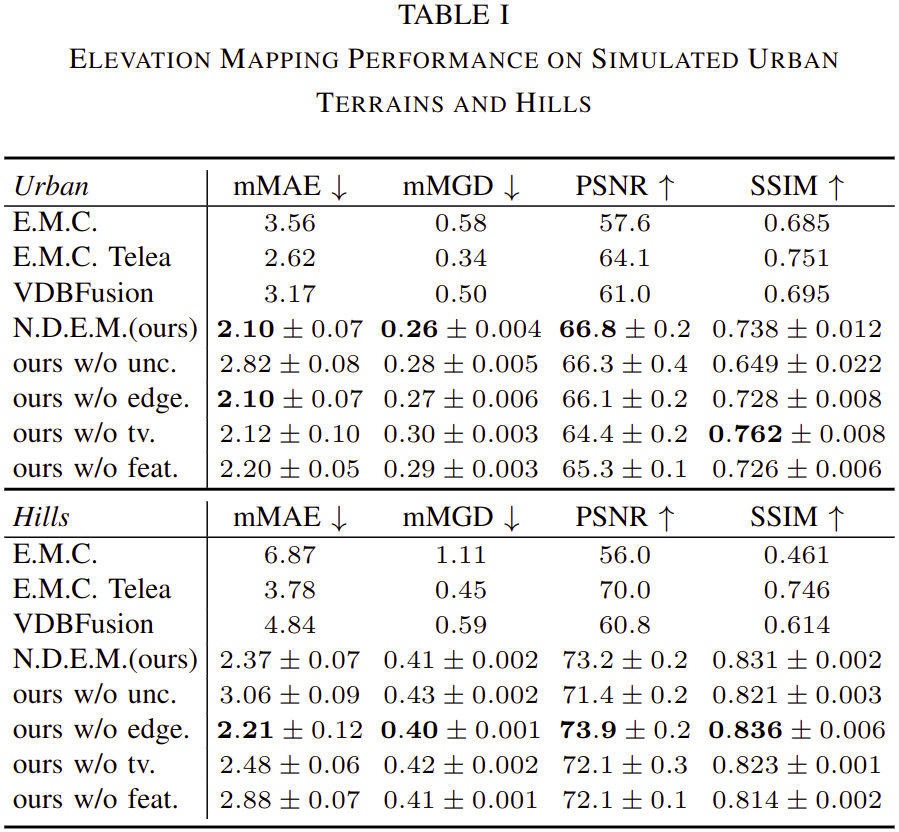
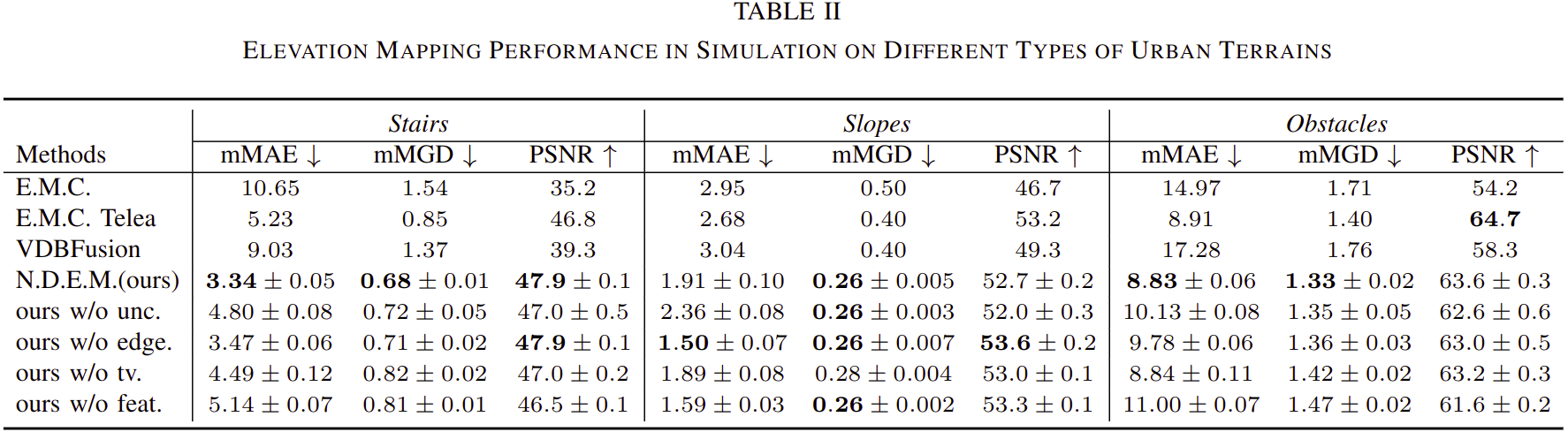
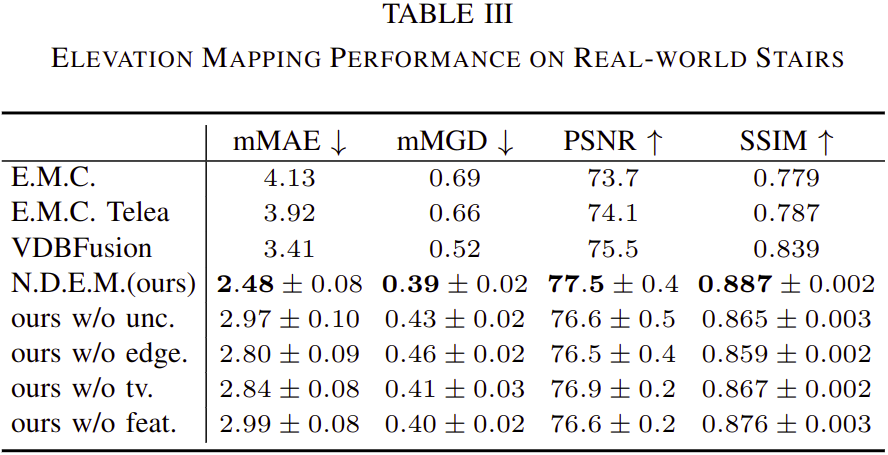
Our framework presents a robust performance in multiple complex scenarios, demonstrating its strong capabilities to provide high-quality elevation mapping service to various robotic applications.